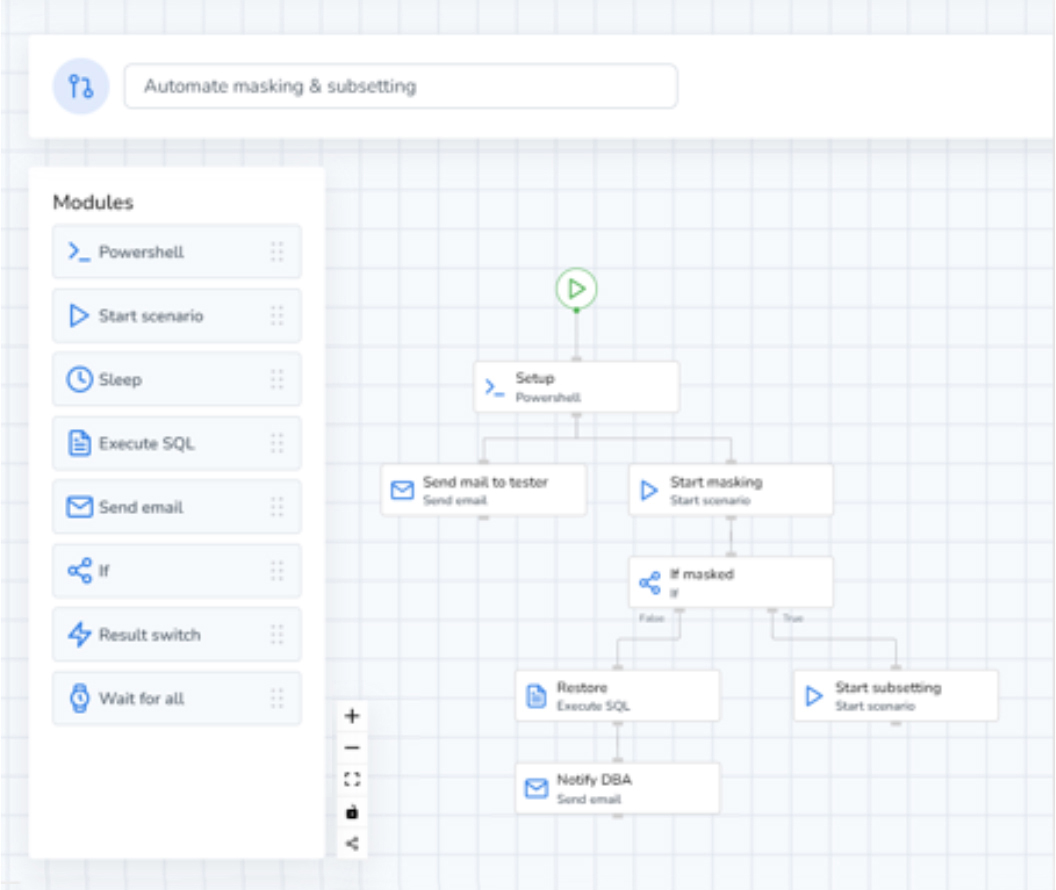
Figure 2 - DATPROF Workflow test data automation pipeline
For starters, DATPROF Runtime – which, as already noted, is the basis of the DATPROF platform – allows you to centrally configure, manage and monitor your test data user-groups, their databases, and the masking and subsetting applications available to them. It also allows you to deliver data to them automatically via DATPROF Workflow, a tool built into DATPROF Runtime that enables you to create automated test data pipelines visually, using a drag-and-drop interface. These pipelines can stretch from test data creation through to the delivery of test data to your testing teams, and are eminently readable and easy to both create and interact with. An example of such a pipeline is shown in Figure 2. Alternatively, a REST API is also provided to do the same job, which may be preferable if you have other CI/CD tools to include in your test data pipelines.
DATPROF Subset is used to create subsets of your existing production data for testing purposes. They are generated using a single, driver table as a starting point, with other tables included based on their relationship to that table. These relationships can be derived from existing database relationships or specified manually, and the process is assisted by intelligent suggestions for which table content should be included in full, as opposed to in part. The results can be visualised as either a data or process model. These are helpful for understanding your database’s structure, and thus how best to create your subset. Various validation techniques are provided to facilitate this process. Options exist to either completely refresh your test database or to append new test data cases to your existing data content, and duplicate data is handled appropriately while ensuring all constraints remain valid.
DATPROF Privacy is a rule-based data masking solution with native support for Oracle, SQL Server, PostgreSQL, MySQL, IBM Db2 (including Db2 on z/OS) and MariaDB. It can, in theory, support any other data source via a processing engine (which is to say, one of the aforementioned databases) and it can mask data stored in a variety of formats, including CSV and XML. Notably, it masks live data in situ, meaning you never need to move or extract it for the purposes of masking. Masking rules can be customised or leveraged out of the box, and can be applied in a specific order by setting dependencies. The product masks consistently over all of your systems and applications, and it delivers meaningful audit reports on your data masking and subsetting actions. Data profiling (and thus the discovery of sensitive data) is offered through DATPROF Analyze.
DATPROF Privacy also provides the company’s synthetic data generation capability, compatible with all of the data sources listed above. The product provides a selection of replacement data candidates and algorithms out of the box, including logical generators, weighted lists, regular expressions, generators that leverage seed data, and more. You can also build your own, using custom database functions, multi-column seed files (for example, a correlated seed list) and “generator expressions” that allow you to combine other types of generators into a bespoke formula, among other things. Synthetic data is generated directly in the database, in a uniform fashion for all major databases, and either during or after masking depending on whether you want to add data to your subset or replace data that is already there. It is also demonstrably performant, and automatically optimises its process flow to facilitate parallelisation.
More specifically, synthetic data is created against “generation sets” of tables, with each column in the table assigned one of the generators described above. Various configuration options are available for each column, including the percentage of null values to generate. Generated values can be combined to create a fully synthetic data set (for example, concatenating first and last names to get full names), columns can be earmarked to generate simultaneously in order to preserve correlations, and foreign key relationships can be discovered and included in your generated data automatically.
Finally, DATPROF Virtualize, the newest addition to the DATPROF product catalogue, offers database virtualisation, cloning, and snapshotting. This technology allows you to rapidly create personalised, containerised copies – clones – of entire production databases and distribute them to your testers individually. It is possible to do this without consuming huge volumes of disk space (and without breaking the bank on storage costs) because each clone will be very small in size when compared to the original database. This is the case because only the deltas, the way each clone differs from said database, are stored. This technique allows your testers to work with entire databases, rather than subsets or synthetic data sets, if they so choose. This has clear advantages in terms of coverage, although any sensitive data will still require masking. It also allows your testers to modify their test data on an ad hoc basis whenever they feel it is required, since each tester is given their own clone to work with, and to quickly refresh their clone when new test data is available. The product also lets you take snapshots of your test data, which can then be used for performing comparisons (for instance, before and after a test run) and rapid rollbacks.